Discover how customer segmentation and profiling using data science can improve your marketing strategies, drive customer satisfaction, and unlock big opportunities in the market.
December 7, 2023
Big Data Analytics for Customer Segmentation Success
Consider a situation where companies carefully provide discounts to customers who otherwise wouldn’t make a purchase, and deliberately avoid sending marketing messages, such as text messages, to those who have no interest in their promotions. This approach isn’t just a theoretical concept. It’s a practical strategy adopted by businesses that have effectively focused their marketing strategies on categorizing customers through the use of big data analytics
If your company is still relying on sending generic campaigns to your entire database, and your customer data is scattered across multiple systems, the strategies outlined in this article can provide valuable insights into the power of big data analytics. We’ll delve into how leveraging big data and more specifically, data science, can transform your marketing approach from a one-size-fits-all strategy to a refined, impactful customer segmentation model.
Content:
What is Customer Segmentation?
Before we dive into the intricacies of big data and its role in refining marketing strategies, it’s crucial to first define what customer segmentation is. Essentially, customer segmentation involves dividing a company’s customer base into distinct groups, each characterized by shared traits or behaviors. This segmentation is more sophisticated than simple demographic splits — it looks into various dimensions like purchasing patterns, preferences, and engagement levels with the brand.
To better grasp this concept, let’s outline the a few key types of customer segmentation:
Demographic Segmentation: Perhaps the most common form, this type segments customers based on demographic factors like age, gender, income level, and education. It’s useful for targeting products and messages that appeal to specific demographic groups.
Geographic Segmentation: This type involves dividing the market based on geographical boundaries. It’s not just about country or city but can drill down to regions, neighborhoods, or even climate type. Geographic segmentation helps tailor marketing strategies to local preferences and cultural nuances.
Behavioral Segmentation: This type focuses on how customers interact with a brand or product. It includes purchase behavior, spending habits, brand loyalty, and product usage. Behavioral segmentation is key to understanding customer habits and preferences, allowing for highly personalized marketing.
Psychographic Segmentation: Going beyond simple demographics, this type delves into the psychological aspects of consumers, such as lifestyle, values, attitudes, and interests. Psychographic segmentation helps in aligning marketing messages with the intrinsic motivations and desires of different customer groups.
What is Big Data and How Does Data Science Enhance Customer Segmentation?
Big data refers to extensive and complex datasets that are too immense and intricate to be managed and understood using traditional data processing tools such as Excel spreadsheets. In the context of customer segmentation, big data plays a crucial role as the primary source of customer information. It encompasses everything from purchase history and online behavior to demographic details and social interactions.
This is where data science comes into play. Data science is a field dedicated to extracting valuable insights from big data. Data scientists utilize advanced analytical techniques to dissect this vast data source, revealing hidden patterns and insights.
These insights are then used as the building blocks of customer segmentation, allowing businesses to group their customers into distinct categories based on unique characteristics and preferences. Leveraging customer segmentation in data science empowers companies to craft more effective targeted marketing strategies, tailor product offerings, and ultimately enhance customer satisfaction and engagement.
Why Targeted Marketing is More Effective Than Traditional Approaches
Over the past few years, marketing has evolved from mass communication methods (such as generic, untargeted internet banners) to more personalized approaches, such as targeted Facebook ads. Utilizing data science for precise customer segmentation has become an essential aspect of modern marketing strategies. Direct (or targeted) marketing, which focuses on personalized offers derived from customer segmentation, has been shown to be more efficient than generalized approaches.
Consider, for example, a customer who consistently spends $20 every month. Offering them a reward for a purchase of that same amount wouldn’t be an effective incentive, as they are likely to make that purchase regardless. Instead, a more strategic approach could be offering an enhanced reward for purchases over $25, encouraging an increase in their average spending. This type of strategic incentivization, a key element of segmentation in data science, is what makes targeted marketing more impactful.
Today, businesses have access to all the tools necessary for personalizing the entire customer journey across all channels — from SMS and email campaigns, to website interactions, mobile app engagement, and even offline at cash registers or during calls with customer service representatives. The ability to tailor the marketing experience at every touchpoint ensures that customers receive relevant, engaging content that resonates with their individual preferences and behaviors, leading to higher engagement, satisfaction, and ultimately, improved business results.
How Segmentation Helps Companies Increase Profits
Effective customer segmentation hinges on the centralized storage of data from all possible sources — including online stores, landing pages, loyalty programs, ad managers, and various data management and customer relationship management (CRM) systems. The richer and more up-to-date the customer data, the more precise the segmentation becomes, leading to more accurate and impactful customer communications.
Let’s explore how data-driven segmentation and data science are benefitting businesses across different industries.
Increases the Average Order Value (AOV or average purchase value)
There are numerous ways that brands can leverage segmentation to increase their average order value. One example comes from PuffCuff, a haircare and accessories retailer. The brand targets customers who have added less than $50 to their cart with a pop-up, offering free delivery to those who increase their purchase to meet this specified threshold.
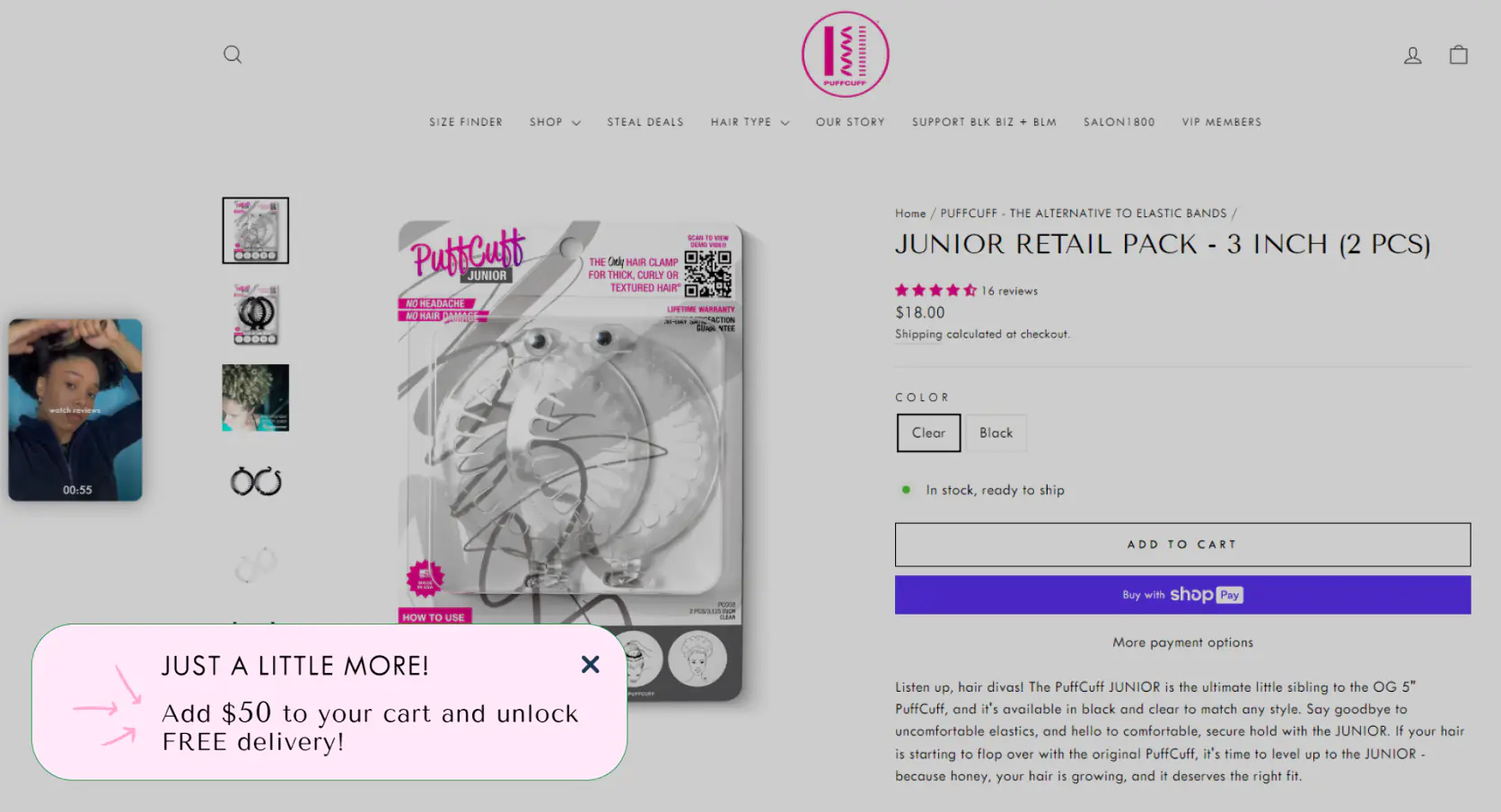
A pop-up offering free delivery, aimed at increasing the average order value of customers who have spent less than $50
Another common tactic is to segment customers based on the types of products they’re considering. If a customer adds a pair of suede shoes to their cart, for instance, the brand might suggest related accessories or shoe care products. This not only tailors the shopping experience to the customer’s current interests, improving their shopping experience, but also increases the potential value of each order.
Boosts Revenue
United Colors of Benetton serves as a prime example of how customer segmentation data science can substantially increase a company’s revenue. In their case, personalized email and SMS campaigns, integral to their omnichannel loyalty program, contribute around 20% to their combined online and offline revenue. Notably, in the online segment, these personalized communications are responsible for up to 32% of the total revenue.
To reliably assess the effectiveness of the loyalty program, Benetton’s marketers have implemented a control group. This group, comprising 10% of their customers, does not receive any marketing communications. The performance and revenue of the main group, which does receive communications, are then compared against this control group. This comparison reveals a significant impact: direct communications to the main group bring in several million in revenue each month.
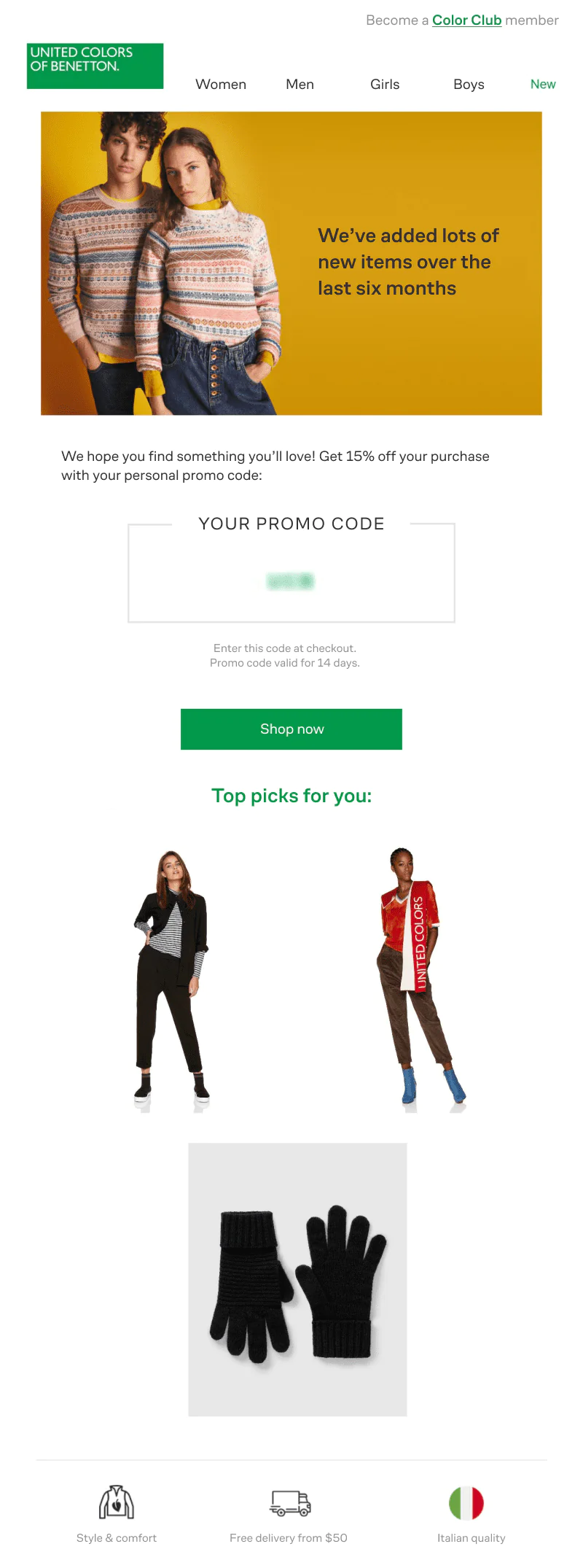
An example of an email targeted at reactivating the churn segment — customers who last placed orders more than 180 days ago
It’s important for us to understand who our customer is, what motivates them to choose us, and what might deter them.This understanding is critical for building and shaping our brand. That’s why, over the past two years, our priority has been to digitize the customer’s interaction with our brand
This knowledge will help us refine the selection and procurement processes for clothing collections, more accurately target our audiences and how we position our products, create communications that speak directly to our audience and provide additional services to club members.
Reduces Communication Costs
Segmentation can help you optimize your marketing expenditures. As an example, Kari, a shoe retailer who uses Maestra to segment their customer base, was able to save an incredible 19% on their marketing budget with the help of effective segmentation. By fine-tuning SMS campaign recipients they were able to exclude 19% of their client base — those who purchased infrequently or who had recently received a special offer.
The primary goal was not just to cut costs, but also to sustain the campaign’s conversion rate. This delicate balance was achieved by ensuring that messages were sent only to those most likely to be interested. As a result, the segmented campaign demonstrated the same conversion rate as the unsegmented SMS broadcast sent to all customers, but with a 19.5% lower cost per order.
Increases Website Conversion Rates
Blossom Flower’s targeted use of lead capture pop-ups serves as a great example of how effective segmentation can enhance website conversion rates. By introducing two pop-ups — one appearing after a visitor browses several pages and another when they show signs of leaving the site — they successfully capture leads at critical engagement points. This approach has allowed them to collect 7,836 leads within just 29 days after the pop-ups were launched.
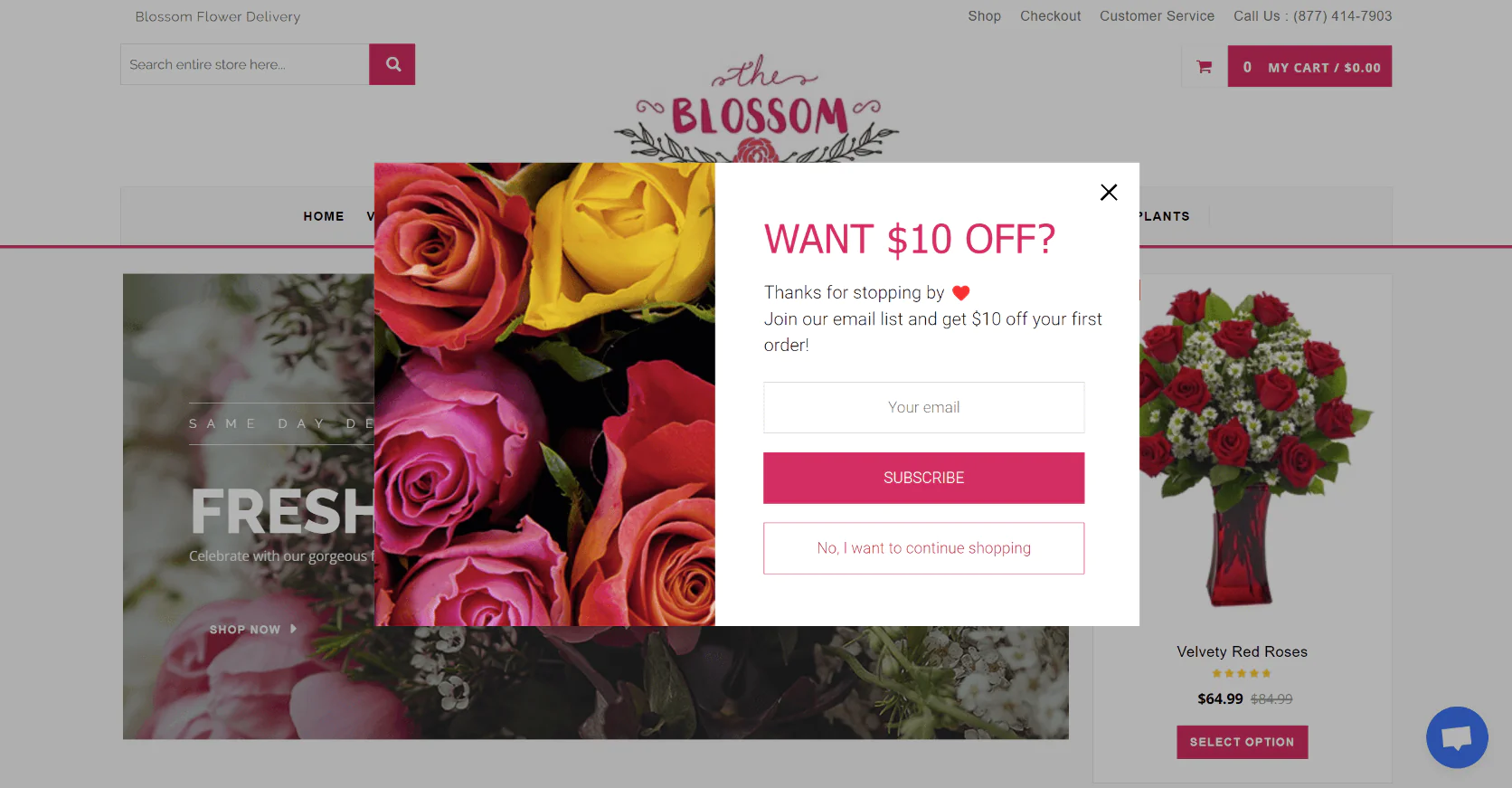
Blossom’s most successful lead capture pop-up, with a 9.81% conversion rate
When Segmentation Might Be Less Effective
Effective customer segmentation relies on several key factors, and its success can be limited in certain circumstances:
Scattered customer data
If customer data is stored in separate systems — such as offline purchases in one system, email interactions in another, and abandoned cart information on the website — then segmentation will be fragmented. For instance, a customer who regularly buys online may mistakenly be classified as a lost customer due to their inactivity in the offline data system.
Un-deduplicated customer base.
Effective segmentation is hindered if the same customer is recorded multiple times in the database under different identifiers. For example, imagine that a marketer wants to target customers with two orders for a discount on their third. If a customer has two profiles, each with one order, they will miss out on this offer despite having made two purchases.
Absence of a Loyalty Program
For businesses operating both online and offline, the lack of a loyalty program can often mean they only have data about online shoppers. Information about average spend or frequently bought together items will be limited to specific retail locations, not reflecting the behavior of the entire customer network.
Launching personalized campaigns becomes very difficult in this case. If your data doesn’t encompass the entire customer journey across both online and offline platforms, any conclusions drawn about purchasing behavior, frequency, and campaign conversions will likely be based on the online segment. Extrapolating these insights to the entire customer base is problematic, as online and offline behaviors can differ significantly.
Delayed Data Updates
This is especially critical for products with a high purchase frequency, like groceries or diapers. If the customer database is updated less frequently than the rate of purchases, the resulting segments won’t accurately reflect the current scenario. Customers might receive outdated messages or unnecessary discounts.
Recognizing the Right Time to Implement Data Science Segmentation
Determining the right moment to integrate data science-driven segmentation into your business strategy is crucial. Here are key indicators that it’s time to make this transition:
You’ve exceeded your growth potential. If your company is seeking to increase revenue, average transaction value, purchase frequency, or other key performance indicators, and traditional mass marketing methods like generic promotions and email blasts are no longer effective, it’s time to switch to personalized strategies.
Declining marginality. If marketers are solely running broad promotions like "20% off everything" and find that these offers are mainly utilized by customers who would have made purchases even without the discount incentives, it signifies a need for change. These blanket promotions erode profit margins without providing clear insights into their real effectiveness.
Customer communication channels are chosen haphazardly, without any reliance on data. When you have no strategic plan over customer communication channels, effectiveness suffers. For example, if you may find that you’re investing a lot of effort (and money) into targeting unresponsive customers via the SMS channel — with the help of data science, you would be able to determine which customers respond well to text campaigns and which customers should be targeted using other channels.
Marketing hypotheses are not tested. Verifying hypotheses is a crucial part of understanding customer preferences and the foundation of personalized marketing. For instance, one of Maestra’s clients, a grocery chain, used A/B testing to determine whether customers preferred discounts in monetary value or percentage. The result was a significant 80% better conversion rate for percentage discounts.
For such tests to be reliable, it’s essential to have a sample that is segmented correctly. In our client’s test, the selected customers had made at least three orders historically but none in the last 45 days. If test groups are not segmented properly (and without big data, this becomes a much more challenging task), the conclusions that are drawn may be inaccurate, and therefore A/B tests become less effective.
Resource-intensive manual database management. If marketers are struggling to create segments manually, drowning in extensive Excel files, and IT or analytics departments are constantly overstretched, it’s a sign to automate segmentation processes. Manual data transfers, like weekly uploads of contact lists and opt-outs into an email marketing system, are a red flag.
These signs indicate that your business could significantly benefit from integrating big data into your segmentation strategy. Doing so can streamline processes, enhance the accuracy of your marketing efforts, and ultimately, drive better business outcomes.
Implementing Segmentation: Where to Start and Choosing a Technological Solution
Implementing targeted marketing through segmentation requires a systematic approach. Here are five critical stages for a successful implementation:
- Define the implementation goal: Establish a success metric and set expectations for it. The metric should be designed in a way that makes it impossible to feign achievement. A good example of a metric is an increase in revenue and/or margin from targeted campaigns compared to a control group. For example, when implementing Maestra, video editing software company, Movavi, set a clear goal to increase the share of revenue generated by their email campaigns. As a result of the clear, actionable plan that emerged from this goal, the team achieved a 27% increase in the metric they were looking to boost.
- Describe technology use cases: For instance, if a marketer wants to send targeted emails based on RFM (Recency, Frequency, Monetary Value) segments but lacks the resources for analysis, they’ll need technology that can automatically categorize customers into these segments. A great example here is haircare and accessory brand, PuffCuff. Before their Maestra implementation, the team worked with Maestra’s customer success manager to come up with a detailed campaign plan covering different customer lifecycle stages.
- Evaluate the need for integration of customer data: This is essential if your company operates with multiple separate data sources. Without integration, investing in personalization is unlikely to yield results. Effective segmentation requires a holistic view of customer data. United Colors of Benetton, for instance, integrated all customer touchpoints to get the most holistic overview of their client data — this included their online store, email, SMS, and push notifications, as well as offline cash registers at retail stores.
- Assess the speed of implementation and changes: One of the key attributes of personalized marketing is the ability to rapidly adapt and change. The chosen technological solution should support the launch and testing of dozens of new campaigns each month without requiring extensive modifications for each new triggered campaign. For example, Blossom Flower Delivery used Maestra to launch 38 automated campaigns within their first month of working with the platform — this helped the company get a substantial head start on achieving a positive ROI.
- Evaluate the Return on Investment (ROI): This calculation should account for:
- Expected outcomes in terms of the implementation metrics (revenue increase, cost savings, speed).
- Costs associated with the platform and its integration.
- Expenses for staff who will operate the platform.
Jewelry designer German Kabirski collects data using the Last Paid Click attribution model and calculates ROI using the following simple formula:

Each of these stages plays a vital role in the successful deployment of targeted marketing through segmentation in data science. It’s about choosing the right technology that not only fits your current needs but also has the flexibility and scalability to evolve with your marketing strategies.
Recap on Big Data Analytics
Transitioning from traditional, broad-stroke marketing to a more targeted, data-driven approach can significantly enhance revenue, reduce communication costs, and increase website conversion rates.
The journey towards effective segmentation involves clearly defining your goals, understanding the necessity of integrating customer data, and choosing a technological solution that’s agile and adaptable. However, this process is not just about deploying new technology — it’s about embracing a new mindset where every marketing decision is informed by data. It’s also crucial to recognize scenarios where segmentation in data science might be less effective, such as when dealing with disparate customer data or lacking real-time updates.
Ultimately, big data analytics in customer segmentation isn’t just a trend — in today’s marketing landscape, it’s an essential approach that leads businesses to increased customer engagement and profitability and allows them to navigate the complexities of the modern market more effectively.