Discover the power of Machine Learning in ecommerce with these 5 impactful use cases that can improve your business and drive growth.
December 12, 2023
Machine Learning in Ecommerce: Enhancing Customer Experience with AI
Machine learning, or ML, encompasses a range of methods that enable the prediction of a customer’s next move: from calculating the date of their next purchase to suggesting complementary products. ML algorithms search for patterns in customer behavior to base their predictions on.
By leveraging machine learning, global brands like L’Oreal Luxe are able to achieve impactful results such as doubling their CRM communication revenue and drive business expansion. Using examples from our clients, in this article, we’ll delve into how to implement machine learning technologies in e-commerce, ML use cases, and when to expect a return on investment.
What is Machine Learning?
Machine learning, a subset of artificial intelligence (AI), uses algorithms that mimic human cognitive processes (this is also known as “deep learning”). It empowers these algorithms to learn from solving a series of similar tasks, thereby improving their performance and accuracy over time.
Algorithms based on deep learning are widely used in e-commerce. Retailers collect various data about users, such as website browsing activity and purchase histories. Using statistical and probabilistic analysis, ML algorithms decipher the relationships between these data sets to formulate predictions.
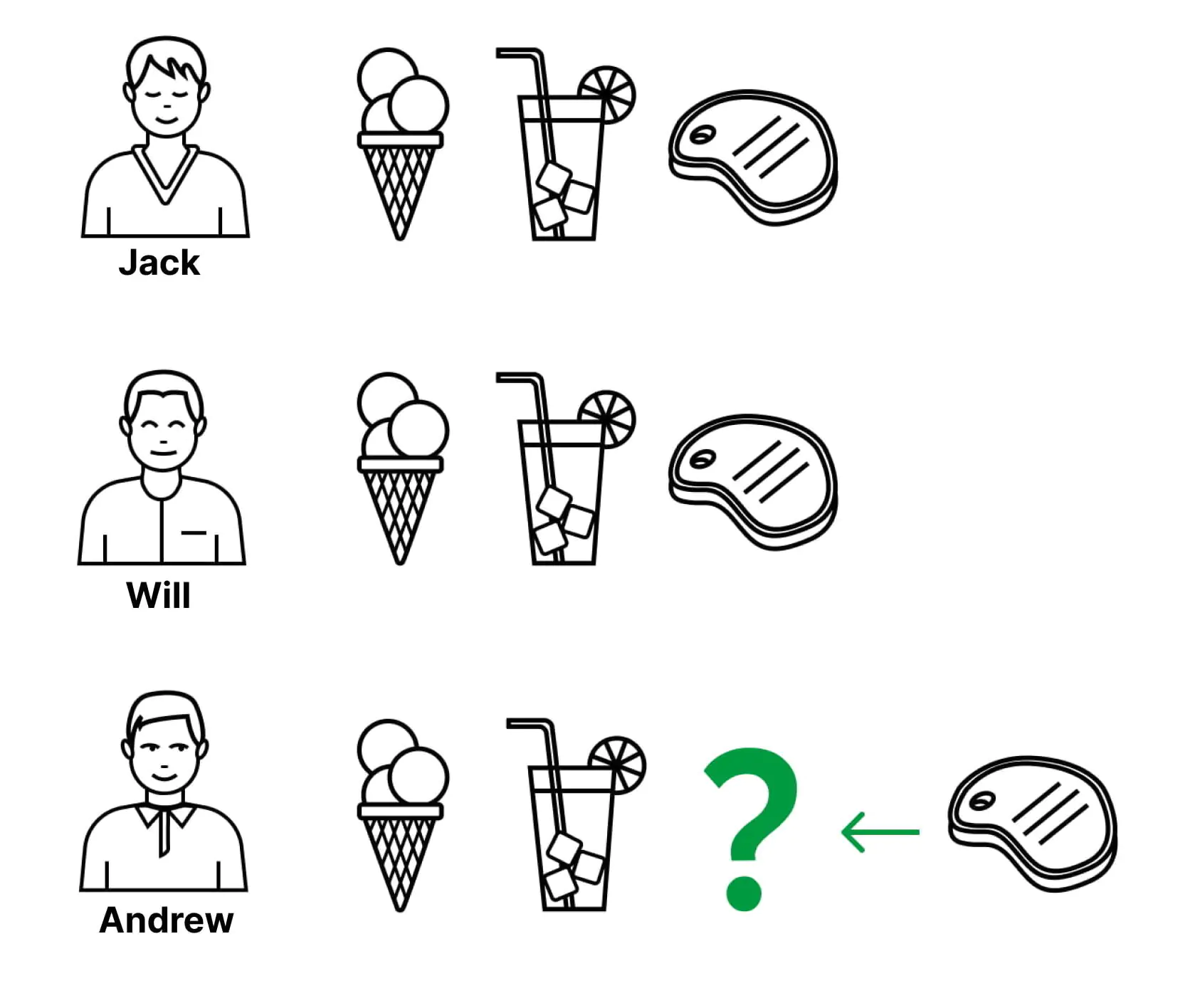
Machine Learning-based algorithms excel at identifying correlations — a basic example would be if two customers both enjoyed a combination of steak, ice cream, and juice, it’s likely a third customer will make a similar choice
The more data that is available for analysis, the more solutions algorithms can provide to various problems. These predictions and solutions are rigorously tested by comparing them against real-world results over time. Predictions that prove accurate are then used as a foundation for further tests. Thanks to their self-improving nature, ML programs continuously enhance their predictive capabilities.
Businesses leverage ML not only to gain a deeper understanding of their customers but also to offer more personalized and relevant recommendations. The key to unlocking the full potential of ML lies in accessing extensive data sets, ranging from search queries to the contents of abandoned shopping carts.
Retailers have begun reaping the benefits of machine learning. With machines processing data, a greater number of parameters are compared, leading to more precise predictions of customer behavior and reducing the likelihood of errors.
For instance, Book24, a book retailer, analyzes customers' browsing and purchase histories to optimize its email campaigns. By implementing ML algorithms, the company can predict the best time to send newsletters to individual customers, rather than sending bulk campaigns. As a result of this approach, the brand was able to achieve the following results:
- Open rate increased by 25%.
- Click rate increased by 20%.
- Conversion rate doubled.
- Unsubscribe rate decreased threefold.
An illustrative example of ML’s impact in the food industry is Burger King’s implementation of a loyalty program based on machine learning. By analyzing historical purchase data, their AI system was able to make personalized recommendations to customers, suggesting items they might like. This approach led to a doubling of customer lifetime value (LTV) and a substantial increase in monthly profits from upselling.
Machine Learning Use Cases
Here are some of the key use cases where machine learning is making a significant impact in the ecommerce realm.
Recommendation Engine
In most ecommerce companies knowing what to recommend to customers at what point can be difficult — this is where machine learning can help through the use of recommendation engines. These systems can perform various roles, from suggesting products to recommending complementary items and adjusting prices dynamically. Product recommendation engines are crucial in enhancing customer engagement, increasing the average order value (AOV), and sales as a whole.
The success of these engines lies in their ability to personalize the shopping experience. By analyzing user data like browsing patterns and purchase history, machine learning tailors product recommendations for individual customers and segments them into distinct groups automatically. This level of customization ensures customers are presented with items that align closely with their interests.
Personalization
Deep learning in e-commerce allows for a level of personalization that goes beyond product suggestions to customize the entire browsing and shopping experience. By analyzing a mix of structured and unstructured data, including images and text, machine learning identifies customer patterns, tailoring content on websites and apps to increase engagement and conversions.
Ecommerce machine learning algorithms consider various factors like style preferences, color choices, browsing history, and individual likes and dislikes. The result is an experience uniquely adapted to each user, making their interaction personally relevant.
Smart Search
Personalized search experiences, enabled by machine learning, adapt to both immediate and long-term user preferences, offering more accurate and tailored search results than standard methods. With a rich dataset, machine learning fine-tunes search outcomes based on factors like preferences, location, search history patterns, and purchase history, leading to products that match better with user needs. This results in higher click-through and conversion rates, as well as increased average order values.
Price Optimization
Machine learning algorithms automatically adjust prices based on factors like:
- Supply and demand.
- Competitor pricing.
- Historical data.
- Season.
- Location (for different currencies, for instance).
Machine learning algorithms instantly evaluate all of these elements (and more), ensuring customers see the most relevant and personalized pricing information.
Prediction
Predictive analysis provides deep insights into various aspects of customer behavior and market trends, such as:
Purchase Predictions. This aspect of predictive analytics focuses on understanding and anticipating future buying patterns of customers. By analyzing historical purchase data, browsing habits, and other relevant customer interactions, machine learning algorithms can forecast what products customers are likely to buy in the future (and when). This insight allows businesses to tailor their marketing and product offerings more effectively, ensuring they meet the evolving needs and preferences of their customers.
Customer Churn Predictions. Identifying customers who are at risk of ending their relationship with the business is another critical area where predictive analytics makes a significant impact. Machine learning models analyze customer engagement levels, purchase frequency, and satisfaction indicators to pinpoint those who might churn — armed with this information, ecommerce companies can develop targeted retention strategies, offering personalized incentives (such as promo codes for inactive customers) or addressing potential issues proactively to maintain a loyal and engaged audience.
Demand Forecasting. Accurate prediction of product demand is essential for efficient inventory management. Predictive analytics uses machine learning to analyze market trends, seasonal variations, and consumer behavior patterns to forecast future demand for products. This level of precision in demand forecasting helps businesses optimize their inventory levels, reducing the risk of overstocking or stockouts, and ensuring that they can meet customer demand promptly and efficiently.
Fraud Prevention
Deep learning significantly improves fraud prevention in e-commerce by reducing false declines and enhancing the customer experience. Unlike manual transaction reviews, which are slow and prone to errors, machine learning operates in real-time, accurately scrutinizing transaction data to distinguish between legitimate and fraudulent patterns that could signify potential security breaches. This efficiency not only speeds up the checkout process but also maintains customer trust and satisfaction.
How Machine Learning Helps Enhance Sales: Real Life Examples
Forecasting Demand and Sales Volume
AI analyzes dependencies, such as how demand changes with the season, price, and product popularity, to predict which products will be high in demand.
For example, Auchan, a big-box store, uses AI for inventory management and optimal pricing. They developed an ML algorithm for demand forecasting, based on past analytics, to plan purchases and reduce costs. The company estimated that implementing this algorithm increased revenue by 2% and reduced excess inventory by 5%.
Boosting Revenue Through Personalization
AI suggests products that customers are most likely to buy. Recommendation systems display these products to increase the average purchase value and frequency.
For example, INCANTO, a lingerie and swimwear brand, used ML to create collections of related products, displayed via widgets on their website. This approach resulted in a 5.5% increase in website revenue.
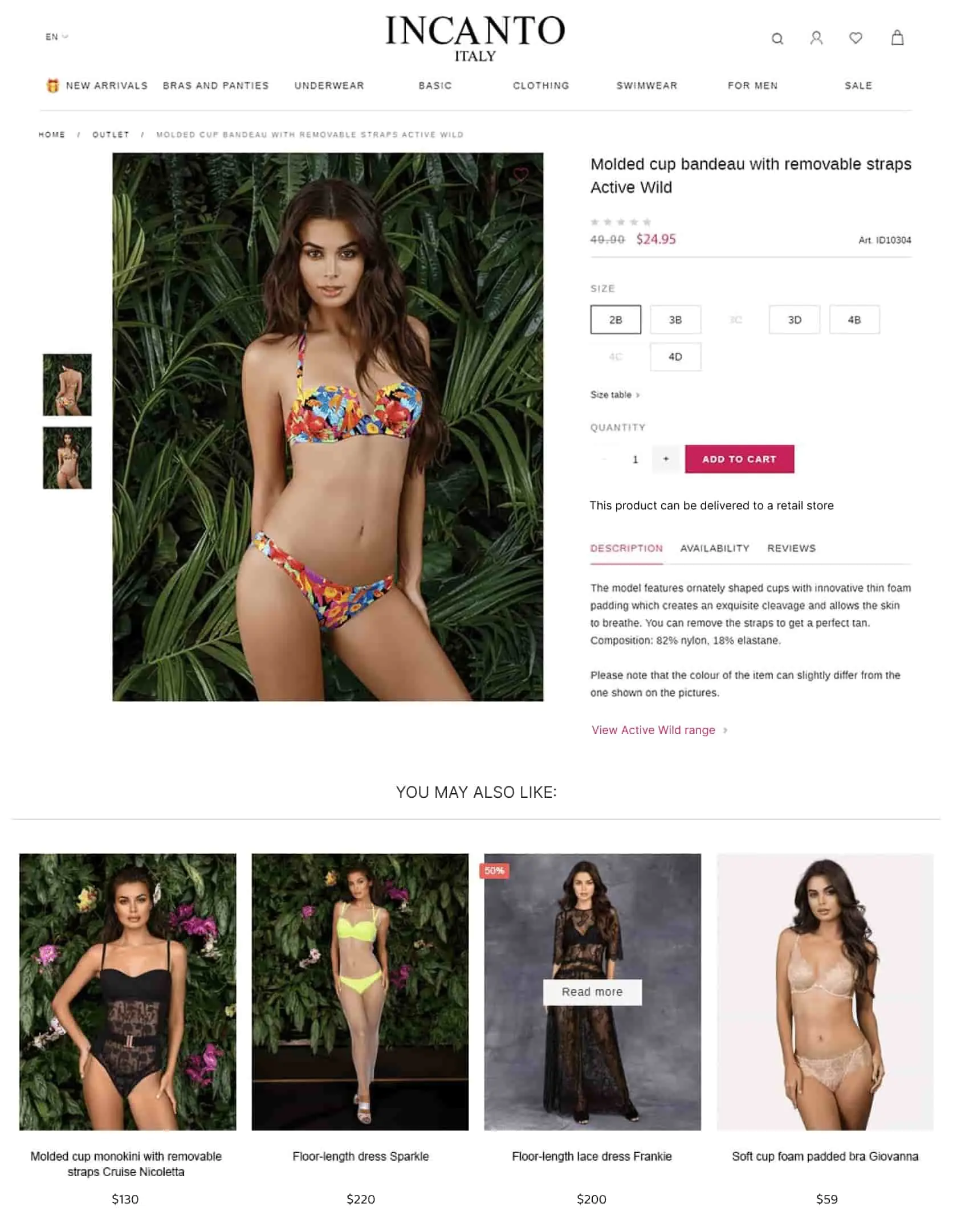
Recommendation widgets suggest more products the customer may be interested in buying. This generates an additional 5.5% revenue from the website for INCANTO
Gaining Insights Without Direct Customer Interaction
ML algorithms collect information from users' digital footprints, analyzing site viewing patterns and responses to company emails. This data provides invaluable insights for detailed customer profiles.
Walmart, for example, uses AI algorithms on its website and app. Shoppers can virtually try on clothes by uploading a photo or selecting a model similar to their height, body shape, and skin tone in the app.
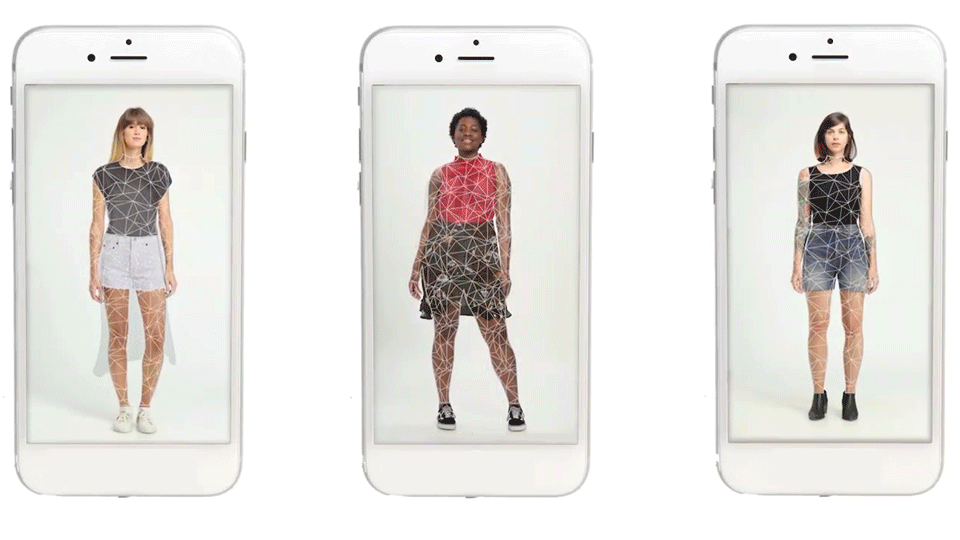
Walmart’s virtual try-on helps customers visualize what different items would look like on them without having to visit a store in-person
Another common example is live customer support chats, where routine tasks are entrusted to chatbots. These operate around the clock to answer questions and human consultants only intervene when the bot is unable to assist. This approach streamlines customer service, ensuring quick responses to common queries while freeing up human resources for more complex issues.
Optimizing Marketing and Advertising Budget
The better a company knows its customers, the more relevant content it can offer — for example, more targeted ad banners or sending discount coupons to those ready to buy. This reduces spending on ineffective marketing and promotions.
Haircare and accessory brand PuffCuff uses Maestra’s “Next Best Action” (NBA) algorithm for personalized product recommendations. The system predicts when a customer is most likely to make a purchase and triggers the NBA marketing flow, which sends an email containing personalized recommendations for each recipient.
A classic goal with machine learning is predicting a user’s likelihood of converting into an order. At Maestra, we have implemented this in our “Next Best Action” (also known as “Next Best Offer”) algorithm, which aims to provide the optimal offer for a customer’s next purchase.
In modeling this algorithm, factors like a user’s purchase history, website visits, and interactions with newsletters (opens and clicks) are taken into account. These data points help us determine the most probable time for an email to successfully lead to a sale.
Spadream, an online premium cosmetics store, conducted an experiment to test the impact of product recommendation widgets on conversion rates.
The audience was divided into two groups: one group was shown the recommendation widgets, while the other wasn’t. After 17 days, the team discovered that customers exposed to product recommendations made 20% more orders compared to those who weren’t.
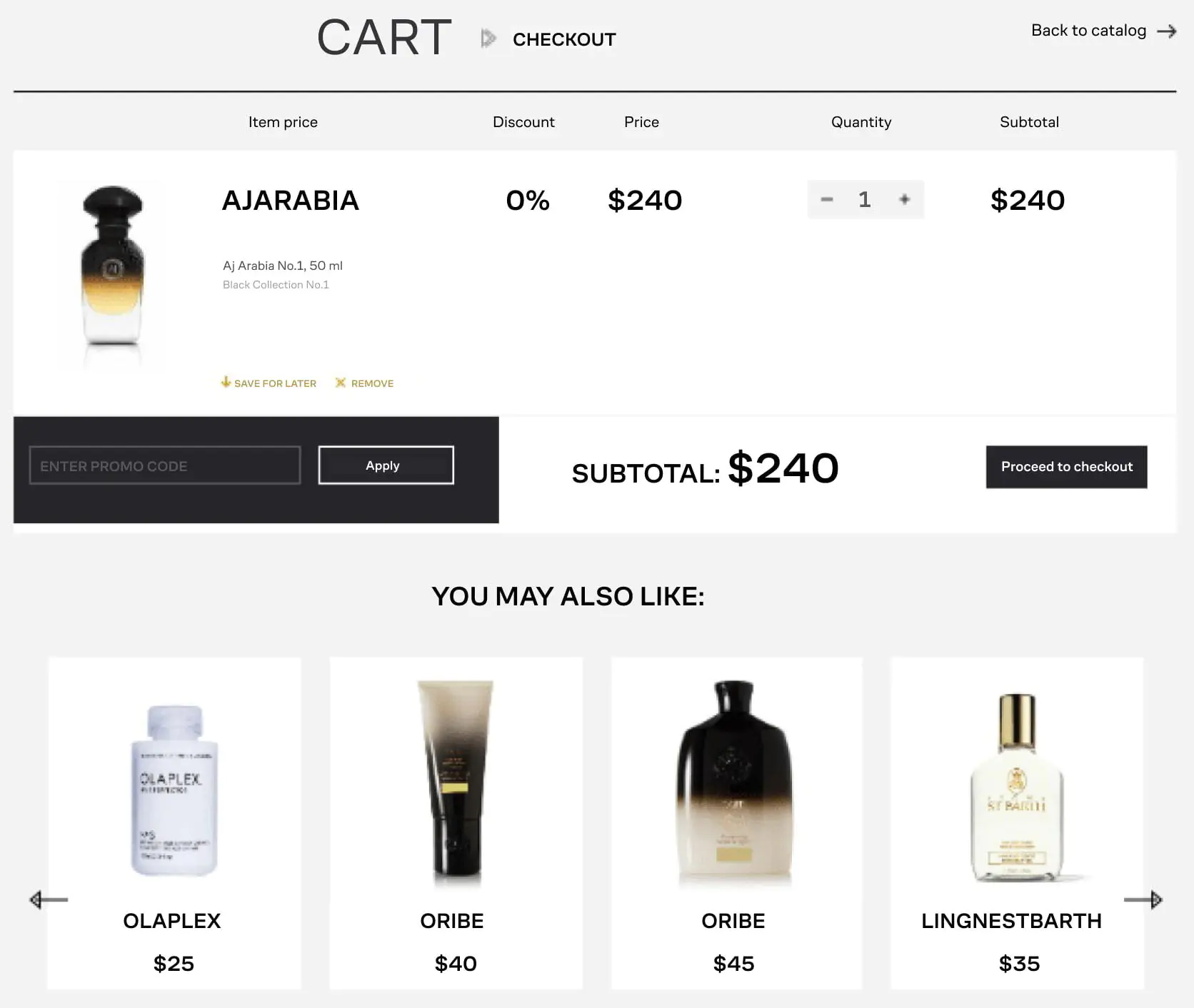
Product recommendations are generated based on the customer’s purchase history over the last 30 days
Increasing Audience Loyalty
ML-based algorithms help build long-term relationships with customers, increasing retention rates. They identify inactive customers post-purchase and segment them for targeted reminders in newsletters and push notifications.
Maestra offers different inbuilt ML algorithms to enhance loyalty enhancement and reactivate customers — this includes algorithms such as "Related Products" and the aforementioned "Next Best Action."
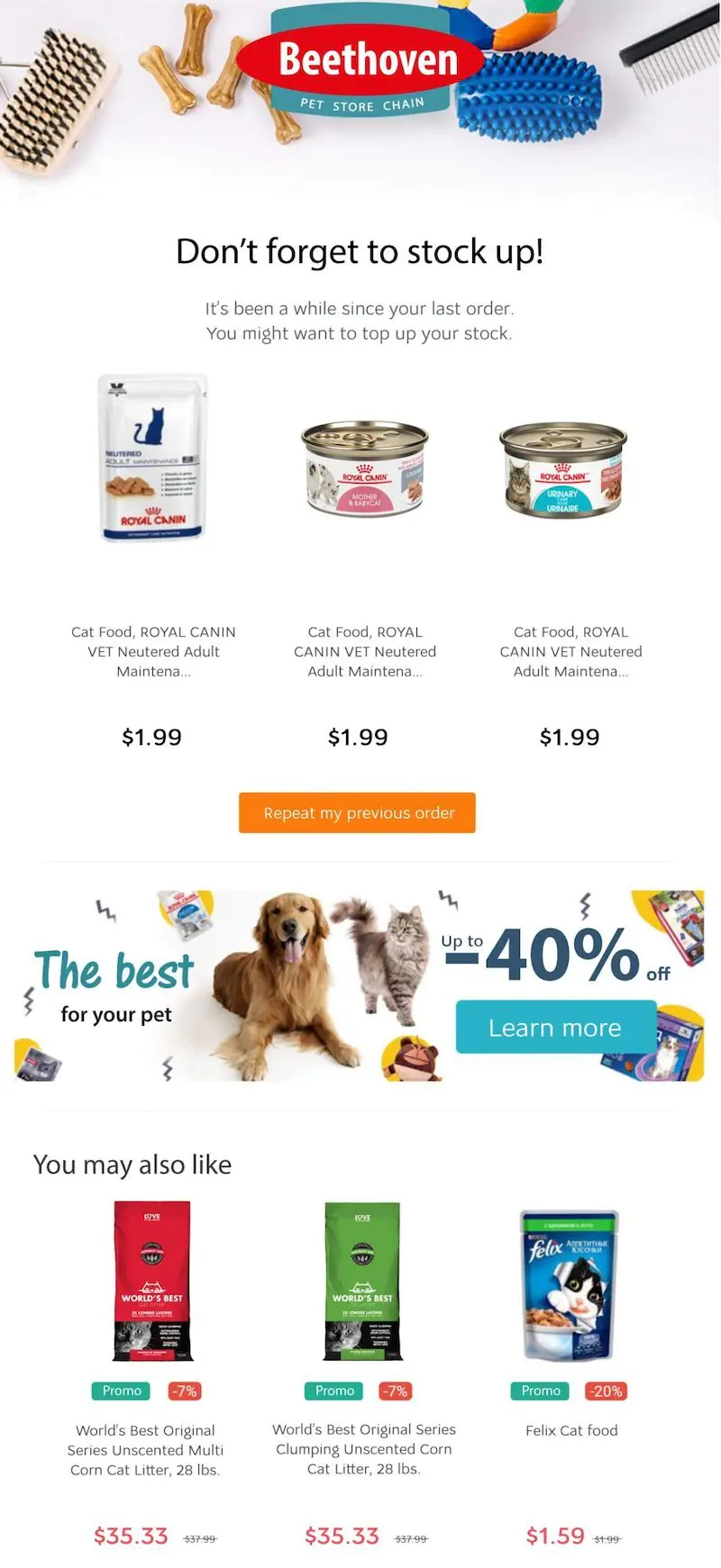
Beethoven’s customers are sent an email with a “Repeat My Previous Order” button when the system predicts the customer is about to run out of goods they previously ordered — this is achieved through the Next Best Action machine learning algorithm
Another key algorithm is “Best Sending Time” which determines the time each customer is more likely to open and engage with an email newsletter. This algorithm tailors the sending time based on individual customer habits. For example, if one customer typically opens emails at 10 AM on Mondays, while another does so at 4 PM, the newsletters are sent accordingly to maximize engagement.
For fashion retailer 12 STOREEZ the highest engagement rates occur on Mondays, with an average open rate of 19.6% and a click rate of 2.9%. The algorithm incorporates this insight, scheduling the company’s newsletters for Mondays to capitalize on these peak engagement times.
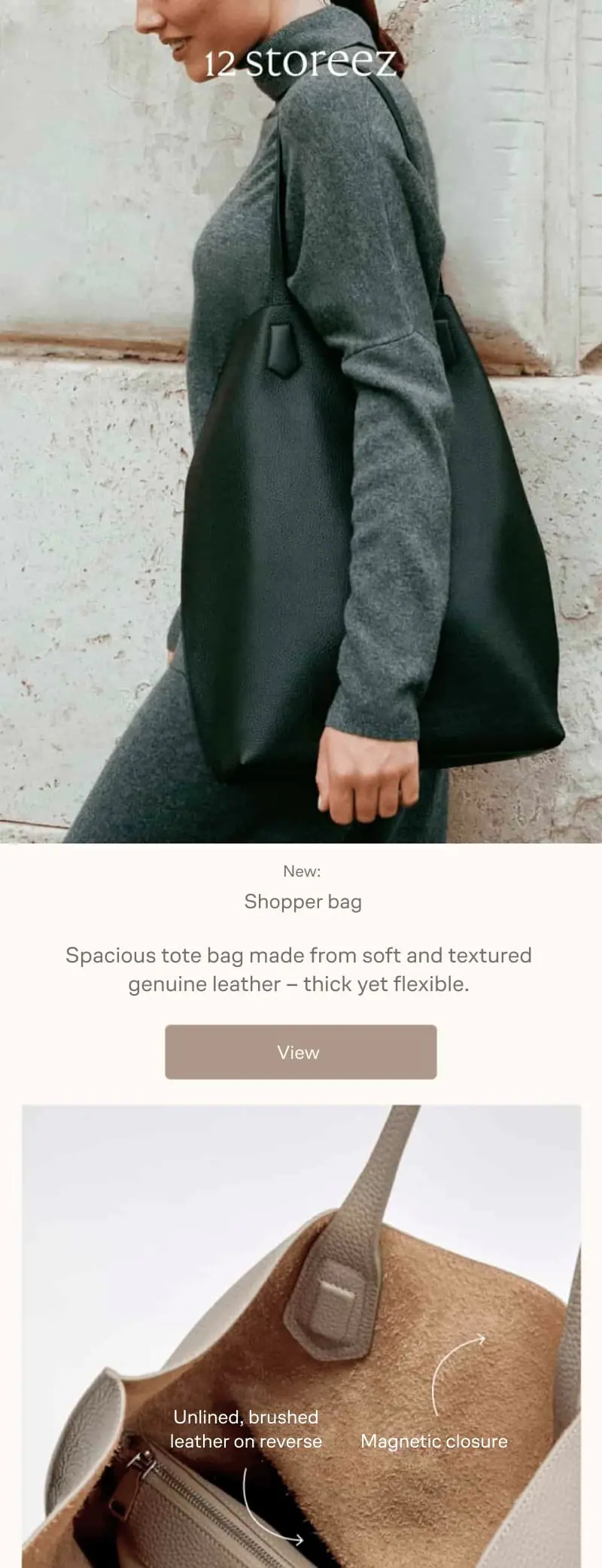
12 STOREEZ’s customers demonstrate more campaign engagement and activity on Mondays, with higher open rates and clicks on website links
Results from A/B tests with a control group revealed that newsletters based on ML algorithm recommendations lead to a decrease in customer unsubscribes and an increase in open rates, click rates, and conversion rates.
Ignoring individual subscriber preferences and sending blanket newsletters to everyone at the same time can result in losing large chunks of your audience
Improving Logistics and Offline Retail Management
Beyond digital applications, AI also aids in planning efficient delivery routes and streamlining warehouse operations, such as order assembly.
Walmart’s “Intelligent Retail Lab” uses computer vision technology to process and analyze images and videos. Sensors and cameras track product stock levels and customer activity, and the program sends inventory replenishment requests to the warehouse when needed.
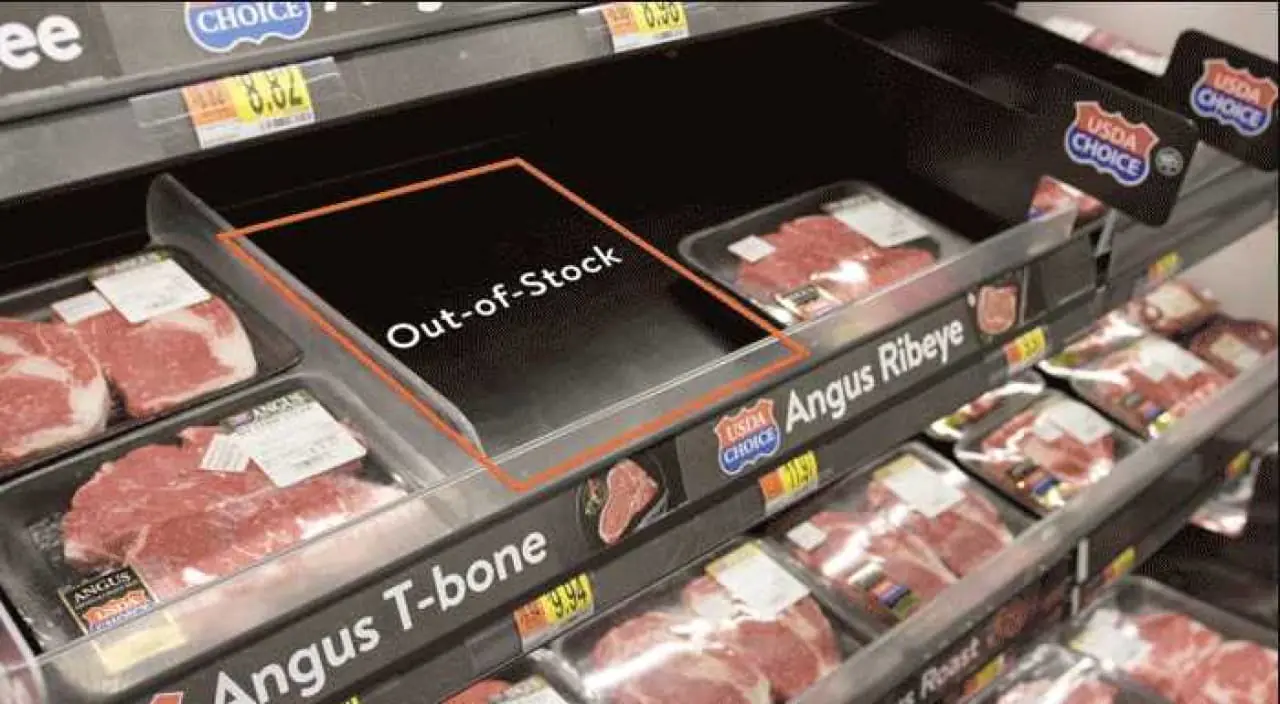
Computer vision sensors monitor prices, stock levels, and product expiry dates
Driving Expansion
The retail industry is a prime candidate for adopting AI technologies due to the abundance of customer and product data available. This data enables quick changes in customer engagement strategies, efficient ROI assessment, and smarter marketing decisions.
H& M Group, a leading global fashion company, has scaled up to 5.000 stores in 72 countries, significantly aided by AI technology. Since 2016, the brand has been deploying AI algorithms to meticulously analyze sales receipts and returns, varying by country and season. This data-driven approach ensures efficient stocking of stores and aims to maximize sales without resorting to discounts.
Improving Customer Satisfaction
One of H& M Group’s brands, Weekday, piloted an AI body scan program which used machine learning to individually tailor jeans for customers. A special device scanned the customer’s body in 3D and modeled jeans specifically for them. Within a few weeks, Weekday produced a custom pair of jeans for that customer. By the end of the pilot, 80% of customers were pleased with their customized jeans. Implementing more ecommerce machine learning technologies like this could help increase customer satisfaction and reduce the percentage of items returned.
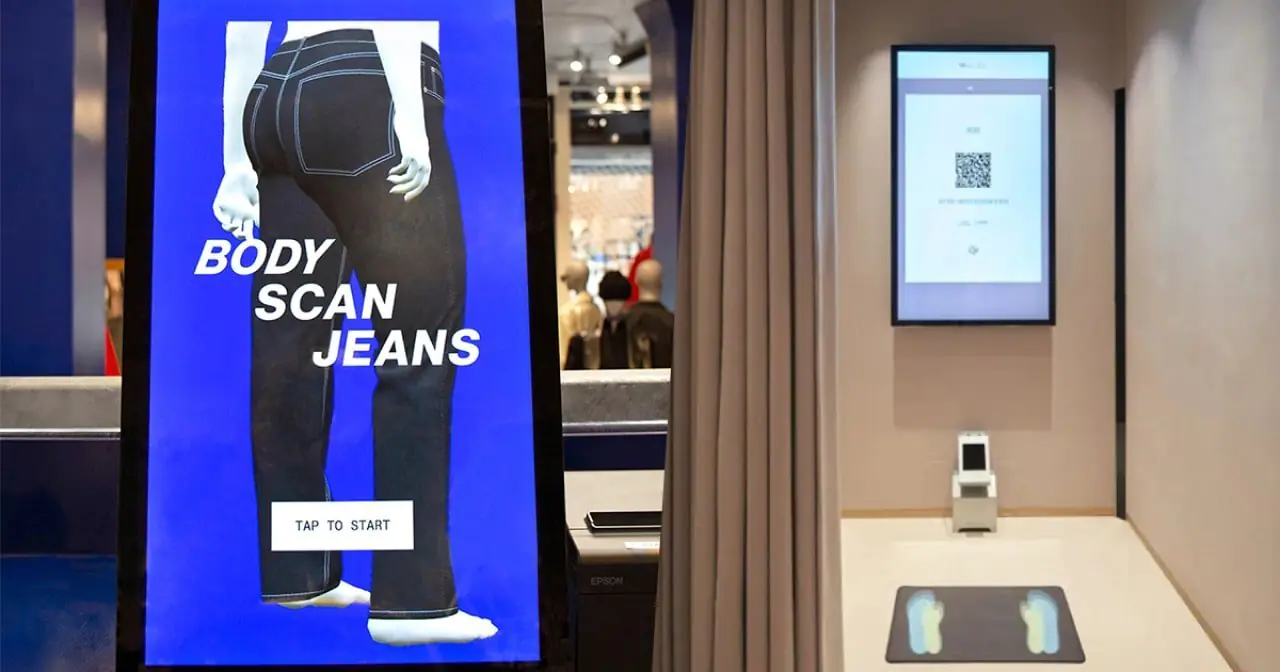
Weekday’s customers were able to scan their measurements in a fitting room, and the program suggested suitable styles, taking into account fit, color, and length
When to Consider Implementing ML Algorithms
Machine learning algorithms are most beneficial for companies that have access to extensive user data from sales and customer interactions. For newer companies or those with limited data, it takes longer to train the algorithm and receive accurate predictions.
Launching ML algorithms in new projects is not advisable if there’s no history of user interactions, particularly for recommendation algorithms. For instance, to predict the best sending time for email newsletters, an understanding of previous email engagement times is essential. If the data shows that most emails are read during lunch breaks, this could form a basis for a testable hypothesis.
To train the mathematical model for an algorithm, we take data from each user’s action history: page views, average time spent on the website, etc. We analyze purchase frequency and how the customer responds to emails. Based on this, we test various hypotheses, like what changes if we alter the frequency of newsletters or email sending time.
However, using ML can be worthwhile even with a small number of newsletters or a low open rate. The most significant results from ML algorithms will emerge later, once there’s enough historical data on customer actions and improvements have been made to the newsletter content.
Most of our clients can test the “Best Sending Time” algorithm within 2-4 weeks post-implementation. On average, that’s how long it takes to achieve a statistically significant test result. For other algorithms, the testing time depends on the campaign in question.
Developing custom algorithms for processing large data sets can be challenging and costly, especially for large companies. It is often quicker and more effective to integrate with a service where these challenges have already been addressed.
Maestra assists various industries, including retail, in implementing ML to enhance communications with users, through personalized product recommendations and marketing campaigns.
Conclusion
The integration of Machine Learning (ML) and AI in e-commerce and retail has emerged as a game-changer, offering businesses the tools to revolutionize their customer engagement and sales strategies. As seen with companies like Walmart, H& M, Incanto, and others, ML enables a deeper understanding of customer preferences, optimizes inventory management, and personalizes the shopping experience. The success of ML lies in its ability to process vast amounts of data, revealing patterns and insights that are invaluable for business growth.
While the journey to Machine Learning implementation may be more challenging for newer companies or those with limited data, the long-term benefits of ML are undeniable. From improving marketing strategies and customer engagement to streamlining logistics and inventory management, the applications of machine learning are broad and impactful.
Ultimately, ML is not just an asset but a necessity for staying ahead in the competitive landscape of e-commerce and retail. It enables businesses to not only understand and meet customer needs more effectively but also to innovate and adapt in an increasingly data-driven market.