Key Figures +25 % additional revenue generated by recommendations +$0.85 increase in revenue per session, according to Google Analytics It took 3 Google Optimize tests to find a successful version of the recommendations What Was Done and What Were the Results Olant has now unified recommendations in mailings and on the website. We set up the transfer of sales from online and offline to a single CDP. […]
Product Recommendations Generate +25% of Revenue for the Olant Mom & Baby Store
The following case study is from Mindbox, the original brand behind Maestra’s technology
Olant is both an online store and a network of stores in Eastern Europe, for mothers, newborns, and children under the age of three. Olant has 200,000 customers in its database, an ERP-based website, and retail solutions.
Previously, Olant had already used Mindbox product recommendations for mailings. However, they used another service for product recommendations on their website. Olant decided to switch completely to Mindbox to make the recommendations homogeneous in the email channel and on the website.
In the process, they wanted to settle all the previous issues, including taking offline sales into account in the recommendations and learning to change the composition of the recommendations at their discretion, which should ultimately allow for the efficiency to be analyzed. Sergey Gerdov, Product Manager at Olant, shares his opinion about the work carried out.
Key Figures
-
+25 %additional revenue generated by recommendations
-
+$0.85increase in revenue per session, according to Google Analytics
-
3Google Optimize tests to find a successful version of the recommendations
What Was Done and What Were the Results
Olant has now unified recommendations in mailings and on the website. We set up the transfer of sales from online and offline to a single CDP. We recommend products based on the customer’s purchases both online and in retail stores. At the same time, we can quickly adjust the settings, for example, and advise a particular brand as a priority.
We compared the efficiency of the three options for product cards on the website. Option 1: we do not recommend anything. Option 2: we recommend similar and related products. Option 3: we recommend only related products. The second option led the way. Two months of testing showed +25% revenue relative to the control group, which didn’t receive any recommendations. That’s $0.85 more for each session.
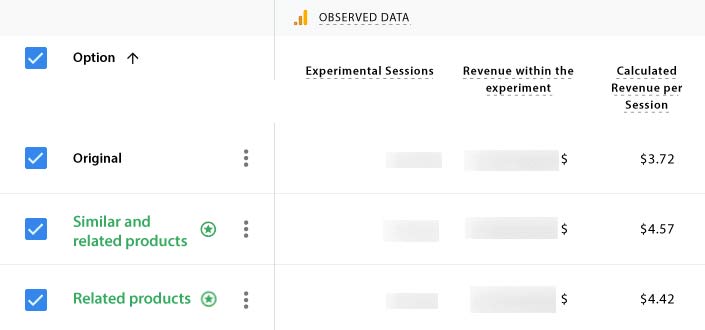
We had been using Mindbox for our email marketing for 5 years when we decided that it was time to try website recommendations. With this, we were looking to improve our online store’s KPIs. We were using another software provider at the time, but Mindbox proved to be more client-focused and had better results. What’s more, the cost of the Recommendations module was lower than our subscription fee for the other service. Phil and Igor were great on this project and we’re happy with the results.
Starting Hypotheses
In fact, the starting hypothesis used to be the standard accepted truth: product recommendations in the product card produce additional revenue.
What Was Done
To run product recommendations on the website and measure the efficiency, we carried out the following steps:
- We integrated the online store and retail sales into a single CDP and set up a cross-product identification, so the same product in the online and offline stores now has a single identifier.
- We assembled a block of product recommendations. We created and customized the recommendations, taking into account the wishes of the client.
- We set up a test in Google Optimize and went through three iterations to see the actual result.
We Integrated Online and Offline Sales into a Single CDP
Olant has been using Mindbox for five years for mailings, email product recommendations, and customer segmentation. Previously, the website was the only source of sales data.
We set up the import of retail sales to improve recommendations. Customers are identified by a discount card. Therefore, if a future mother bought a stroller in a retail store in the city center, Olant won’t advise her strollers in mailings or on the website.
The ERP system unloads orders for the last day via FTP once within a 24-hour period, then Mindbox CDP automatically receives sales data. We set up a cross-product identification in order for CDP to understand that the stroller from the website and the stroller from the brick-and-mortar store are the same product.
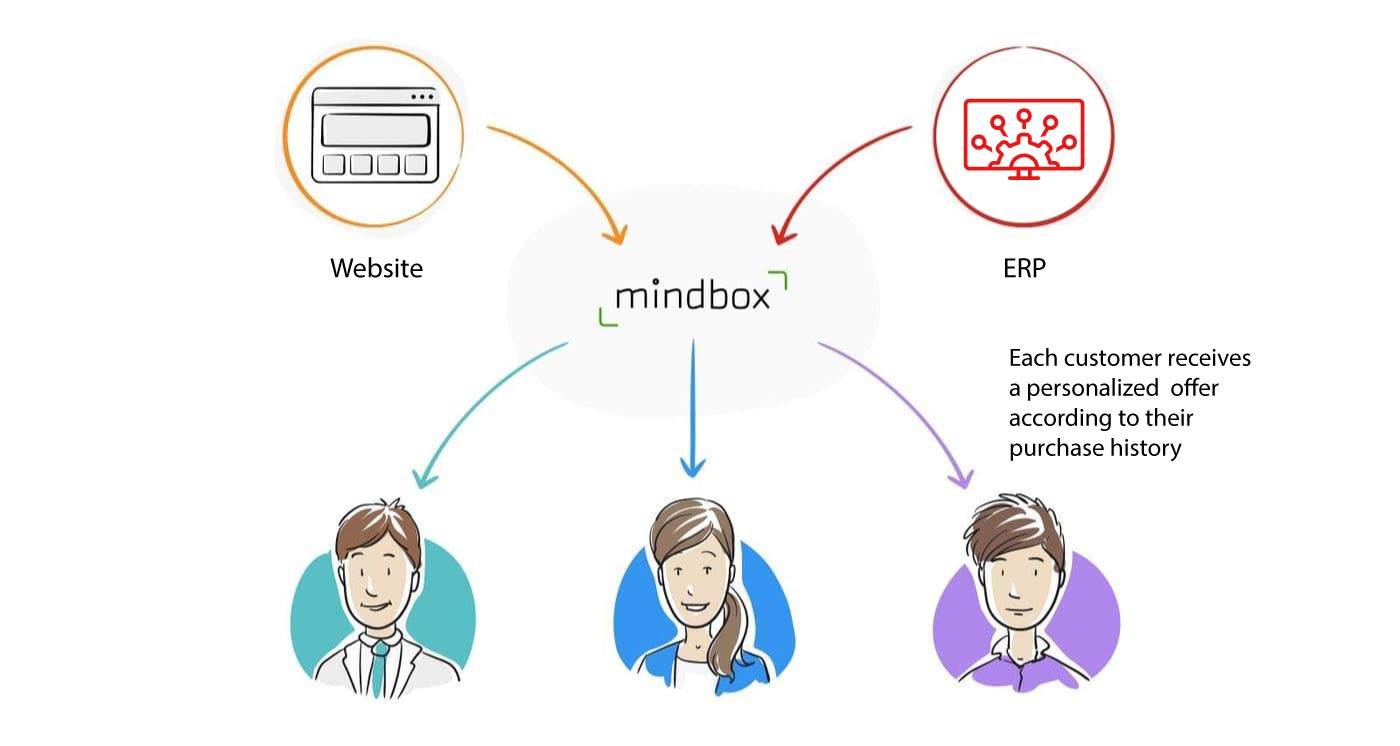
Mindbox keeps a complete history of customer purchases both from the online and offline segments. We will never advise a customer a product that they have already bought.
We Assembled a Block of Product Recommendations by Laying It Out and Configuring All the Parameters.
Product Block Layout
Olanta’s product recommendation widget allows the customer to add a product to favorites or to the cart, and to see its availability in brick-and-mortar stores. It took five days to configure the complete layout and iron out all the bugs.
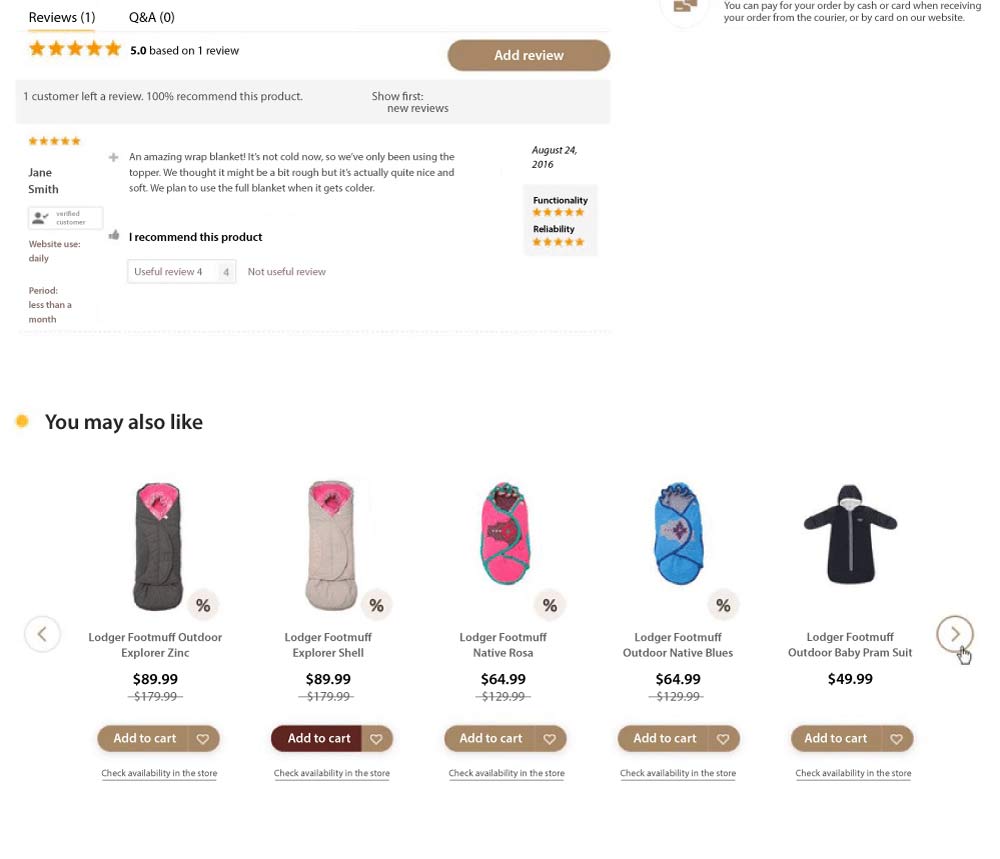
You can pay for your order by cash or card when receiving your order from the courier, or by card on our website
Configuring Recommendation Rules
In the Mindbox interface, we set the rules for displaying recommendations and configured them to Olanta’s liking. For example, it was important for Olanta that the Munchkin brand was advised in the nutrition section first and only then the others.
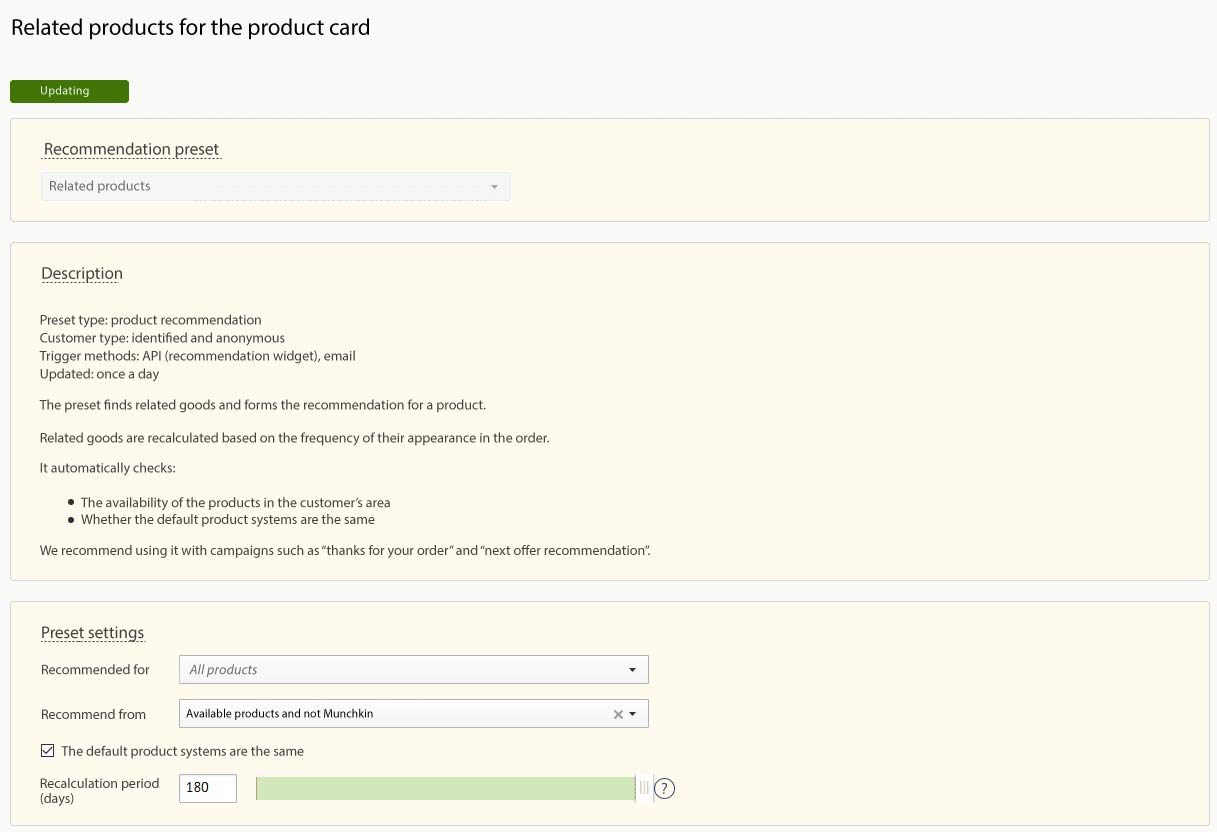
Related product settings
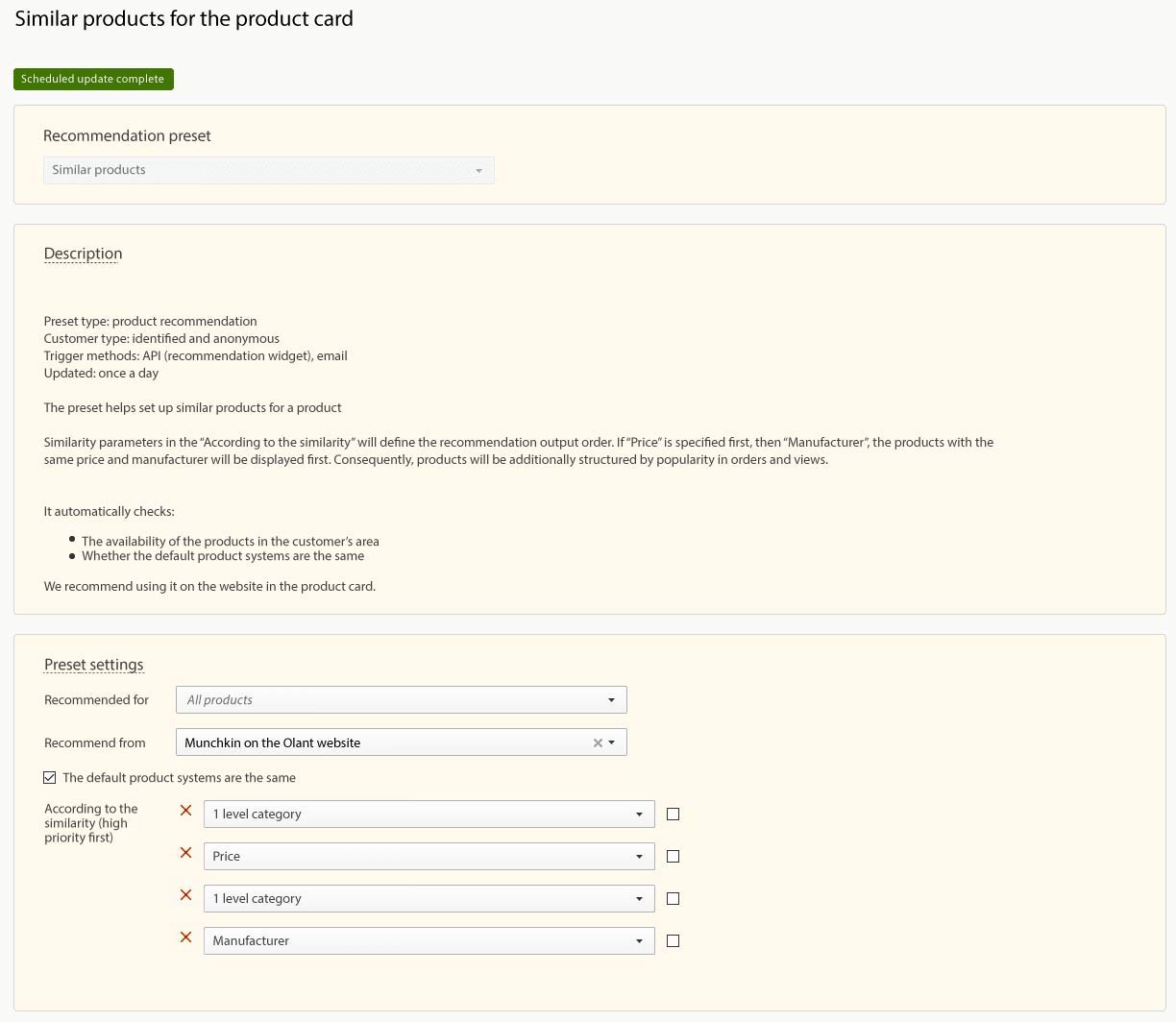
Similar product settings
Setting Up a Test in Google Optimize
To measure efficiency, we set up a test in Google Optimize. It took three tests to see the actual result.
First Test
We set up the first test by comparing a card without recommendations (the control group), and with a card that recommended similar and related products inside. The recommendations were inferior by a large margin.
We began to look into the reasons behind it and found the “add to cart” button wasn’t functioning. Without this button, it is impossible to assess the efficiency of the recommendations. We fixed the button and ran the second test. We learned an important lesson: we shouldn’t wait for the test to be completed, it’s better to check it in the process to see whether everything is going according to plan.
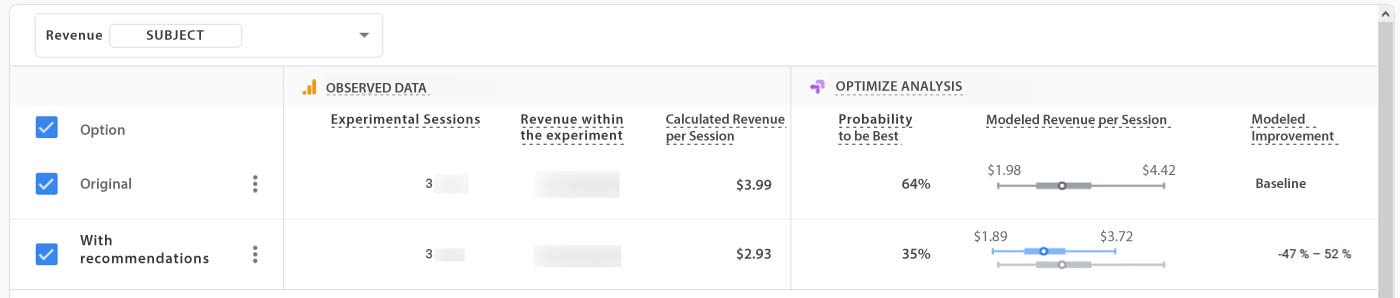
Second Test
Even after the bug was fixed, recommendation revenue was ~50% behind the control group. The version featuring recommendations had more viewed items, but less revenue.
The failure was explained by the fact that the recommendations covered similar cheap products. The customer was switching to these products and buying them rather than the expensive original option. In the case of the card without a recommendation, the customer settled on the first expensive option, seeing no alternatives.
We turned off the second test and changed the parameters that allowed us to determine similar products. We increased the price indicators to advise similarly priced items to customers.
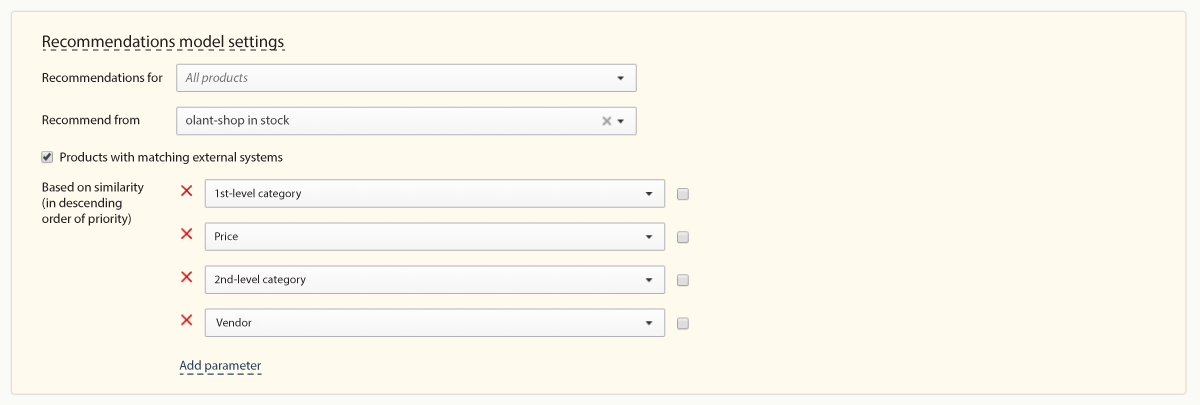
Third Test
In the third test (no bugs and with new settings for similar products) we altered another significant parameter, adding a third option. The first option was the control group. The second option included recommendations regarding similar and related products. The third option is based only on related products.
The third iteration of the test showed statistically significant revenue. It’s curious that the option that included only related products brought in a smaller amount of revenue than the option with similar and related products. Each session in the version with related and similar products brings $0.85 morethan without them.
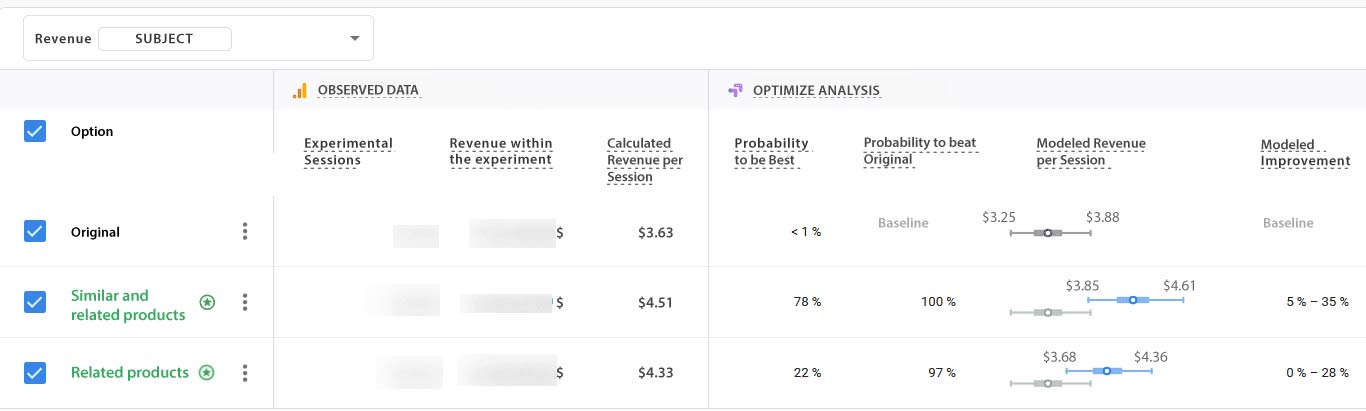
Conclusion
In two months, Olant had its website product recommendations laid out, set up, implemented, and tested, which ultimately led to statistically significant revenue. The implementation of product recommendations increased website revenue by 25% or $0.85 per session, according to Google Analytics, relative to the control group.
Moreover, we took offline sales into account, adjusted the campaigns according to their objectives, and made the recommendations on the website and in email uniform.